by Shruti Joshi and Rakhe P. Balachandran^ India recently codified the 29 Central labour laws into four labour codes viz., code on wages, industrial relations, social security, and occupational safety, health and working conditions. The code on industrial relations has also introduced fixed term employment (FTE). This study synthesises the international evidence on the impact of FTE using a meta-regression analysis. Based on 19 studies done in different country contexts, we find that FTEs, on an average, have a positive impact on labour productivity. Labour laws in India have been shaped by the recommendations of various national committees and commissions including the first national commission on labour (1969), the national commission on rural labour (1991), the second national commission on labour (2002), and the national commission for enterprise in the unorganised sector (2009). These committees recommended reforms on social security, wages, insurance, and industrial relations. Many of these changes have been added in a piecemeal manner, making the labour laws complicated. Recently, India codified the 29 Central labour laws into four labour codes viz., code on wages, industrial relations, social security, and occupational safety, health and working conditions. The code on industrial relations aims to consolidate the laws relating to trade unions and settlement of industrial disputes. This code subsumed three erstwhile central labour laws: Trade Unions Act, 1926; Industrial Employment (Standing Orders) Act, 1946, and Industrial Disputes Act, 1947. Provisions of this code include timely settlement of disputes, flexibility to hire fixed term workers and limiting sudden disruption in work due to strikes and lockdowns. It revamped the structural procedure for negotiation of labour disputes and introduced FTEs in India, which is supposed to make labour management more flexible. FTE is viewed as a mechanism to enhance labour flexibility according to the business cycles, which may lead to overall economic efficiency, albeit available evidence points towards mixed impact of FTE on labour productivity. FTEs are popular in European countries for increasing firms’ flexibility of labour management. FTE employees are entitled to same pay, allowances, and benefits like the permanent workers1 and are directly hired by firms by avoiding middlemen2. However, FTE employees are not entitled to any termination notice or severance pay and any retrenchment benefit because of non-renewal of their contract. This imparts flexibility of labour management without incurring any additional redundancy costs. On the one hand, such flexibility enables firms to meet seasonal fluctuations in demand, but being temporary in nature, it demotivates workers. Employers may also not have the incentive to provide on-the-job training to these employees, thus, adversely affecting their productivity (Arulampalam and Booth 1998; Booth et al. 2002; Fourage et al. 2012; Vidal and Trigges 2009). Alternatively, FTE can also act as a screening mechanism to identify productive workers by firms and would lead to overall improvement in labour productivity (Wang and Weiss 1988; Gerfin et al. 2005; Gash 2008). How do we draw inferences about the impact of FTE for India on labour productivity from these studies? Toward this purpose, we conduct a meta-analysis of 19 studies to comment on what India can expect on the labour productivity front, from the implementation of the industrial relation code which introduced FTE. With this backdrop, the remaining article is organised as follows: Section II provides an overview of the literature analysing the impact of FTE on growth, employment and productivity. Section III explains the construction of Meta data. Section IV explains the meta-regression model, section V provides results and VI concludes. II. Literature Review The strand of literature analysing the relationship among labour regulations and economic indicators mostly relate employment protection legislation to economic growth, productivity and employment. There is evidence in the literature that flexible labour laws enhance industrial output (Holmes 1988; Besley and Burgess 2004; Sanyal and Menon 2005; Ahasan and Pages 2009). Onerous labour regulations, on the other hand, adversely affect the growth prospects of economies (Caballero et al 2004; Micco and Pages 2006). A meta regression on 53 studies reveals that only 28 per cent of studies agreed with the IMF consensus that labour market deregulation increases employment and reduces unemployment (Brancaccio 2020). Further, Author et al. (2007) find that mandated employment protection reduces capital deepening and firm productivity. Labour market regulations, including minimum wages and mandated severance pay, impact unemployment by reducing the demand for labour (Lazear 1990; Currie and Fallick 1996; Abowd Kramarz and Margoliz 1999; Blanchard and Wolfers 2000; Heckman and Pages 2003; Kugler 2004; Boetero et al 2004). Minimum wages create a floor on wages and constrain the ability of employers to freely decide wages, thus, adversely impacting the employment generation. However a meta regression, on 236 estimated minimum wage elasticity and 710 partial correlation coefficients from 16 studies, examining the adverse employment effect of minimum wages in the UK found that minimum wages do not have any adverse employment effect (Leonard et al 2020). Many studies examine the effect of FTE on productivity (Sanchez et al 2000; Ortega et al 2010; Lucidi 2010). The human capital theory argues that employers are reluctant to invest in firm-specific human capital and training on temporary employees due to their short duration, leading to a tradeoff between FTE and investment in human capital (Arulampalam and Booth 1998; Booth et al. 2002; Fourage et al. 2012). Second, substitution of core workers by fixed term workers reduces the motivation of both core and fixed term workers (Vidal and Trigges 2009). Thirdly, high level of FTE can stimulate sectoral shift in activity, as usually low productive sectors benefit the most from temporary employment (OECD 2005). On the other hand, in the presence of imperfect markets and asymmetric information, FTE enables firms to screen new workers, hence, incentivising workers to put in efforts. Firms which offer permanent contracts to most productive workers can improve their overall productivity (Wang and Weiss 1988; Gerfin et al. 2005; Gash 2008). The impact of temporary contracts on total factor productivity is reported as negative in the literature (Dolado et 2008; Addessi 2011; Hospido et al 2015). Studies in the Indian context, in general, point to a positive relationship between flexibility in labour laws with industrial employment and output. To illustrate, in States with rigid laws, there was a slowdown in output, employment and productivity (Besley and Burgess 2004); industries located in states with flexible labor laws grew more quickly in response to liberalization policies (Aghion et al 2003); industrial employment was more sensitive to shocks in labor market in states with flexible labor laws (Sharma et al 2011); increased cost of labor disputes reduced the output and employment in registered manufacturing (Ahsan and Pages 2008). III. Data This study focuses on the estimated impact of FTE on labour productivity. The article search for the meta happened during April-June, 2022. We mainly used four search engines, viz., google scholar, ECONLIT, Science Direct and JSTOR. In addition to these search engines, we also referred to the reference list mentioned in the identified studies from the first round of search. Keywords for the search included various combination of ‘fixed term employment’ ‘temporary employment’, ‘labour contract’, ‘fixed term contract’, ‘temporary contract’, ‘productivity’ and ‘labour productivity’. Based on the above search, we were able to identify 65 studies. To be added in the meta, a study must contain the empirical estimates of the effect of FTE on productivity. Two theoretical studies which did not contain empirical estimate were not included in the meta. Further, 39 studies did not study the impact of FTE on productivity, and, hence, were removed from the meta-analysis. These studies were looking at the impact of employment protection legislation on productivity instead of FTE. Lastly, two studies were purely descriptive. This process led to the elimination of 43 studies out of the identified 65 studies. Further, we have also excluded three studies on total factor productivity. As a result, we selected 19 relevant studies with 311 coefficients for meta-analysis. In all the included studies, the variable on FTE was the proportion of temporary/fixed term workers to the total workers. Two forms of theoretical specification exist for measuring the dependent variable: partial measure and total factor productivity (TFP). We focus on partial measure of productivity. The different partial measures of labour productivity used in the included studies are output per worker, sales per worker and revenue per worker. In addition to this, two studies have used wages and level of production as a measure of productivity. In our analysis, we code per worker measure of productivity as one and zero, otherwise. In establishing the causal relation between labour productivity and FTE, we encounter endogeneity, as shocks to labour productivity can also affect the share of fixed term workers used by the firm. For instance, a negative shock to productivity might induce increased use of temporary workers. Therefore, the effect of FTE on labour productivity is likely to be biased. We have coded studies that have controlled for endogeneity as one and, otherwise zero. The type of data used in the studies also impart variations in the study results. For example, the advantage of using panel data over cross section is that it enables to control for time invariant changes. Thus, the results are relatively more robust from panel data studies as compared to their cross-section counterparts. We have coded studies that used panel data as one and, zero otherwise. We also control the type of survey: coding government survey as one and, zero otherwise. Government surveys have usually wider coverage, structured questionnaire as well as more professional data collection process, making it more reliable and unbiased, as compared to surveys conducted by individual authors. In addition to this, year fixed effects capture any time variant changes which might have occurred during the period of analysis. The country dummy takes value one if the study is based on a single country and zero otherwise. Finally, region control takes the value one if it is a European region and zero otherwise. Other study specific characteristics include firm specific effects such as firm age, firm size, investment, trade union density and sector of economic activity. Firm age and size positively impact labour productivity as these firms have required investments as well as experience which enables them to improve labour productivity. We also control for worker specific characteristics which includes education, type of work and gender. Labour productivity also depends on the nature of work one does; workers engaged in blue collar services will have lower productivity vis-à-vis those involved in white collar jobs. Studies also controlled for other variables such as ownership structure. In some studies, number of children has been used as a measure of productivity for women. Innovation and R&D has a positive impact on labour productivity, which are accounted for by many studies. Further, merger and acquisition are used as an indicator of firm organisation and, performance related pay is a way to incentivise workers to put in more efforts. However, the number of studies that controlled for these characteristics are not high enough to include them in the meta-analysis as moderator variables. Descriptive Statistics Our dataset has 311 observations covering periods from 1987 to 2015 and coming from 7 countries3 and the EU. The largest coming from Italy (35 per cent) and Colombia (24 per cent). All the studies directly report the estimated effect of temporary jobs on labour productivity. There are a couple of studies in the Indian context, albeit, we could not include these studies in our analysis as they were not directly analysing the impact of FTE on labour productivity.4 The distribution of the reported coefficient is shown in figure 1. The range of the reported coefficient is from (-) 0.84 to 1.12. Of the reported coefficients, 60 per cent of the observations are less than zero. This indicates an adverse effect of FTEs on labour productivity while remaining 40 per cent of the observations are more than zero indicating a positive effect of FTE on productivity. None of the reported coefficient is zero which means that the included studies find some effect of FTE on productivity. As can be seen from figure 1, our reported coefficient is bimodal for the entire sample, clearly depicting one mode for the European region estimates and another for the non-European region estimates. The mean effect size of the European region is (-) 0.102 while for the non-European regions is 0.83. (Figure 1 and Table 4). In the meta sample, 73 per cent of the studies are from the European region and 80 per cent of them are single country studies. Seventy per cent of them are published in peer reviewed journals. Majority of the studies have analyzed data from Government surveys (85 per cent) and used panel data (60 per cent). Forty-four per cent of studies have controlled for endogeneity, thus, provides robust results on the relationship between FTE and labour productivity. Studies that controlled for other study specific characteristics such as firm age (14 per cent), firm size (25 per cent), trade union density (20 per cent), investment (19 per cent), education (18 per cent), training (27 per cent), blue collar workers (19 per cent), and gender (14 per cent) are relatively low in the meta-sample (Table 4). 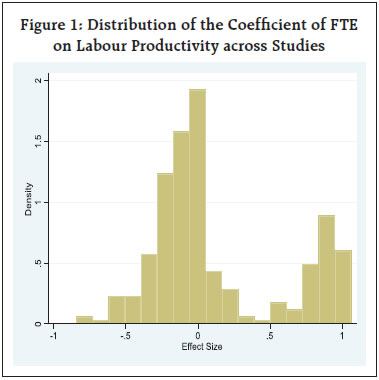 Table 4: Descriptive Statistics | Variable | Code | Whole sample | European Sample | Non-European sample | Total Number of productivity estimates (Dependent variable) | | 311 | 229 | 82 | Average Estimate | | -0.13 | -0.12 | 0.83 | Subjective Measure | | 1.9 | 2.4 | 0 | Per worker measure | | 96 | 95 | 100 | Moderator Variables in Percentages | Data | Panel=1; 0 otherwise | 60 | 81 | 1.2 | Survey | Survey by government=1; 0 otherwise | 85 | 81 | 100 | Estimation | Controls for endogeneity = 1; 0 otherwise | 44 | 27 | 95 | Firm age | Controls for firm age=1; 0 otherwise | 14 | 20 | 0 | Firm size | Controls for firm size=1; 0 otherwise | 25 | 34 | 0 | Trade union density | Controls for trade union density=1; 0 otherwise | 20 | 27 | 0 | Investment | Controls for investment=1; 0 otherwise | 19 | 27 | 0 | Sector of economic activity | Controls for sector of economic activity=1; 0 otherwise | 48 | 66 | 0 | Education | Controlled education= 1; 0 otherwise | 18 | 23 | 0 | Training | Controls for training=1; 0 otherwise | 27 | 36 | 0 | Blue collar | Controls for blue collar worker=1; 0 otherwise | 19 | 27 | 0 | Gender | Controls for gender=1; 0 otherwise | 14 | 20 | 0 | Year Fixed effect | Controls for year fixed effect=1; 0 otherwise | 30 | 41 | 0 | Country | Single country=1; 0 otherwise | 80 | 72 | 100 | Region | European=1; 0 otherwise | 73 | 100 | 0 | Published | Published=1; 0 otherwise | 70 | 58 | 100 | V. Model Meta-analysis refers to statistical analysis of individual studies for the purpose of integrating the findings. It enables researchers to deeply understand what an entire empirical literature implies. Meta-regression is an effective tool to control for the potential publication bias that occurs when researchers tend to report statistically significant findings (Card and Krueger, 1995). To control for the publication bias, every study must report an effect size, viz., coefficient on FTE, and the respective standard errors. As t-statistic is calculated by dividing the coefficient with the respective standard error, when the standard error is high, researcher may search for a large coefficient to establish statistical significance. This will lead to a positive relationship between the coefficient and the standard error. This relationship is modelled in a standard FAT-PET (funnel-asymmetry test- precision effect test) specification to control for publication bias (Stanley and Doucouliagos, 2012). The FAT-PET model depicting the relation between standard error and coefficient on FTE can be represented as follows:  In equation 1, B1SEi is the rough approximation of publication bias. The FAT test is the conventional way to identify the publication bias, H0: B1= 0. After controlling for the potential publication bias, the parameter B0 becomes the unbiased estimate of productivity. Testing H0: B0 = 0 provides a valid method to identify if there is any genuine empirical effect after controlling for publication bias. This is also called the precession effect test. Furthermore, Stanley and Doucouliagos (2014) recommend that if B0 is significant in equation (1), a better approximation of the true effect will be captured using the Precision-effect estimate with standard error (PEESE) estimator which relies on SE squared. In the empirical specification (1), we additionally control for moderator variables. Since the standard errors of each estimate is known, equation 1 can be estimated using weighted least squares (WLS). The productivity estimates are not independent as studies report more than one estimate from the same data. Thus, we use clustered standard error to control for correlation among standard errors (Nelson 2014; Penn and Hu 2018). However, we have only 19 studies, hence 19 clusters, leading to small sample bias in the clustered SE. To address this issue, we use cluster-robust variance estimators with small-sample corrections for linear regression (Tyzlser et al 2017). Table 5: FAT-PET and PEESE Whole Sample | | FAT-PET | PEESE | FAT-PET WLS-corrected | PEESE WLS-corrected | Effect size | Effect size | Effect size | Effect size | S.E. | 0.121 (0.32) | | 0.085 (0.19) | | S.E. square | | -0.722 (-0.58) | | -0.722 (-0.53) | Constant | 0.0852 (0.54) | 0.0905 (0.55) | 0.087 (0.501) | 0.0905 (0.51) | N | 304 | 304 | 304 | 304 | t statistics in parentheses. * p < 0.05, ** p < 0.01, *** p < 0.001. | VI. Results Table 5 gives the FAT-PET and PEESE results for the whole sample without adding the moderator variables. We find that the FAT test indicates no publication bias as the coefficient of SE is not statistically significant. We find that our estimate of unbiased effect of FTE on productivity is also not statistically significant indicating that there is no empirical effect of FTE on productivity. Our results remain the same even after correcting for small sample bias of clustered standard error. Table 6 shows that for the European sample although there is no publication bias, FTE has a marginally significant negative effect on labour productivity. In table 7, we add the other moderator variables. Labour productivity is influenced by a host of variables in addition to the nature of employment contract. Hence, moderator variables are added to equation (1) for avoiding any misspecification bias. After adding the moderator variables, table 7 shows that the average impact of FTE on labour productivity is 0.81, which is significant at 0.1 per cent level in the full sample. In the European sample, the average impact of FTE on labour productivity is marginally significant with a coefficient of 0.85. After correcting for small sample bias of clustered SE, we do not find any significant change in this impact. For instance, for the full sample the coefficient marginally increases to 1.03, whereas for the European sample it marginally falls to 0.7. Thus, meta-analysis of the empirical evidence from countries, who have already implemented FTE for years, shows that FTE has a positive impact on labour productivity, on an average, after controlling for all study specific characteristics. This supports the hypothesis that, in the presence of asymmetric information, FTE enables employers to identify productive workers and it incentivises workers to put in more effort so that they become permanent employees in the firm (Wang and Weiss 1988; Gerfin et al. 2005; Gash 2008). Table 6: FAT-PET and PEESE for European sample | | FAT-PET | PEESE | FAT-PET WLS-corrected | PEESE WLS-corrected | Effect size | Effect size | Effect size | Effect size | SE | -0.0647 (-0.17) | | -0.072 (-0.14) | | SE square | | 0.411 (0.63) | | 0.411 (0.52) | Constant | -0.0838 (-2.11) | -0.0868* (-2.21) | -0.0838* (-1.97) | -0.0868* (-2.08) | N | 226 | 226 | 226 | 226 | t statistics in parentheses. * p < 0.05, ** p < 0.01, *** p < 0.001. | Table 7: Results | | Full Sample | European Sample | Full Sample WLS-corrected | European Sample WLS-corrected | effect size | effect size | effect size | effect size | SE | -1.095** (-3.11) | -1.210** (-3.00) | -1.009** (-3.15) | -1.099** (-2.89) | Type of data | -0.571*** (-4.36) | -0.611*** (-4.11) | -0.571*** (-3.53) | -0.602*** (-3.43) | Survey | – – | -0.326* (-2.51) | | | Estimation | -0.0263 (-1.33) | -0.0212 (-1.06) | -0.025 (-1.25) | -0.02 (-0.99) | Labour productivity (Subjective measure) | -0.0426 (-0.25) | -0.0564 (-0.35) | -0.113 (-0.49) | -0.112 (-0.22) | Per worker measure of labour productivity | 0.00922 (0.93) | 0.00802 (0.84) | 0.007 (0.7) | 0.007 (0.7) | Labour productivity in comparison to other firms | -0.00652 (-0.08) | -0.0170 (-0.21) | | | Published study | 0.0465 (1.07) | 0.0489 (1.23) | -0.024 (-0.3) | -0.021 (0.80) | Firm size | -0.0981 (-2.06) | -0.108* (-2.28) | -0.096 (-1.37) | -0.103 (-1.47) | Firm age | – – | – – | 0.263* (1.45) | 0.292* (1.98) | Trade union density | -0.0127 (-0.77) | -0.00875 (-0.48) | -0.082 (-0.911) | -0.0084 (-0.91) | Sector dummy | -0.0333* (-2.44) | -0.0342* (-2.75) | 0.01 (0.2) | 0.006 (0.12) | Investments | 0.121 (1.54) | 0.119 (1.66) | 0.158 (1.21) | 0.154 (1.28) | Training | -0.580*** (-4.80) | -0.611*** (-4.63) | -0.575** (-3.05) | -0.601** (-3.17) | Education | 0.229** (2.93) | 0.247** (3.04) | 0.206 (2.2) | 0.220 (2.4) | Blue collar | 0.110 (1.49) | 0.108 (1.59) | 0.053 (0.58) | 0.053 (0.58) | Gender | 0.293* (2.51) | – – | | | Country | 0.0273 (1.15) | 0.0327 (1.31) | -0.08 (1.6) | -0.076 (1.52) | Region | -0.329* (-2.14) | – – | -0.302** (-1.68) | | Year | 0.0141 (0.39) | 0.00951 (0.27) | 0.0141 (0.29) | 0.00951 (0.20) | Constant | 0.811*** (14.30) | 0.859* (2.60) | 1.027*** (5.00) | 0.695* (2.07) | N | 304 | 226 | 304 | 226 | t statistics in parentheses. * p < 0.05, ** p < 0.01, *** p < 0.001. | After correcting for small sample bias of clustered SE, we find that in the full sample, other study specific characteristics such as controlling for training and type of data, and the region in which the study is conducted, are also significant determinants of the impact of FTE on labour productivity. The average impact of FTE on labour productivity significantly declines when studies independently controlled for training in their respective estimations. This could be due to the possible positive omitted variable bias created by the variable ‘training’ in studies that did not control for it independently, thus, driving up the average impact of FTE on labour productivity. In some cases, employees on FTE join as trainees or in some cases firms treat FTE as a mechanism to train/screen out their future productive employees (Wang and Weiss 1988; Gerfin et al. 2005; Gash 2008). We find that studies employing panel data reported significantly smaller impact of FTE on labour productivity as compared to studies employing cross section data. This could be due to the higher information content in the panel data in comparison with cross section data. Region affects the relationship between FTE and labour productivity as countries may differ in terms of their market regulations and institutions, thus, influencing this relationship. Conclusion In this study, we employed a meta-regression analysis to understand the impact of FTE on labour productivity based on international experience. On the one hand, FTE can worsen labour productivity as it does not incentivise firms to invest on the fixed term workers; on the other hand, in anticipation of conversion of FTE into permanent contracts, workers can put in more efforts thereby improving their productivity. We synthesize the existing empirical literature on the relationship between FTE on labour productivity to arrive at an unbiased average estimate of this relationship. Our results indicate that FTE has a positive and significant effect on labour productivity. Our meta-analysis showed that controlling for publication bias, the effect size was 1.03 for the entire sample and 0.7 for the European sample. The positive impact of FTE on labour productivity stems from the fact that FTE can function as a screening mechanism to identify productive employees. Moreover, if these contracts are converted into permanent contracts, workers also have the incentive to put in more effort (Wang and Weiss 1988; Gerfin et al. 2005; Gash 2008). Based on the results, the introduction of FTE under the industrial relation code can be expected to improve labour productivity in India, which is currently lower than what is observed in advanced economies. However, the exact magnitude of the impact would be conditional on the specific characteristics of Indian labor market. Studies focusing on India are not currently feasible due to non-availability of data. Therefore, results in this paper provide a broad direction based on international experience to fill this lacuna. References Abowd, J. M., Kramarz, F., & Margolis, D. N. (1999). Minimum wages and employment in France and the United States. Addessi, W. (2011). Labor contracts and productivity dynamics. In XXVI National Conference of Labour Economics, Milan, September (pp. 15-16). Aghion, P., Burgess, R., Redding, S., & Zilibotti, F. (2003). The unequal effects of liberalization: theory and evidence from India. LSE mimeo. London, UK: LSE. Ahsan, A., & Pagés, C. (2009). Are all labor regulations equal? Evidence from Indian manufacturing. Journal of Comparative Economics, 37(1), 62-75. Arulampalam, W., & Booth, A. L. (1998). Training and labour market flexibility: is there a trade-off?. British Journal of Industrial Relations, 36(4), 521-536. Baek, J., & Park, W. (2018). Firms’ adjustments to employment protection legislation: Evidence from South Korea. ILR Review, 71(3), 733-759. Besley, T., & Burgess, R. (2004). Can labor regulation hinder economic performance? Evidence from India. The Quarterly journal of economics, 119(1), 91-134. Blanchard, O., & Wolfers, J. (2000). The role of shocks and institutions in the rise of European unemployment: the aggregate evidence. The Economic Journal, 110(462), C1-C33. Booth, A. L., Francesconi, M., & Frank, J. (2002). Temporary jobs: stepping stones or dead ends?. The economic journal, 112(480), F189-F213. Botero, J. C., Djankov, S., Porta, R. L., Lopez-de-Silanes, F., & Shleifer, A. (2004). The regulation of labor. The Quarterly Journal of Economics, 119(4), 1339-1382. Brancaccio, E., De Cristofaro, F., & Giammetti, R. (2020). A meta-analysis on labour market deregulations and employment performance: no consensus around the IMF-OECD consensus. Review of Political Economy, 32(1), 1-21. Caballero, R. J., Cowan, K., Engel, E., & Micco, A. (2004). Effective labor regulation and microeconomic flexibility. Cappellari, L., Dell’Aringa, C., & Leonardi, M. (2012). Temporary employment, job flows and productivity: A tale of two reforms. The Economic Journal, 122(562), F188-F215. Card, D., & Krueger, A. B. (1995). Time-series minimum-wage studies: a meta-analysis. The American Economic Review, 85(2), 238-243. Castellani, F., Lotti, G., & Obando, N. (2020). Fixed or open-ended? Labor contract and productivity in the Colombian manufacturing sector. Journal of Applied Economics, 23(1), 199-223. Currie, J., & Fallick, B. (1993). The minimum wage and the employment of youth: evidence from the NLSY. de Linde Leonard, M., & Stanley, T. D. (2020). The wages of mothers’ labor: A meta regression analysis. Journal of Marriage and Family, 82(5), 1534-1552. De Linde Leonard, Megan, T. D. Stanley, and Hristos Doucouliagos. “Does the UK minimum wage reduce employment? A meta-regression analysis.” British Journal of Industrial Relations 52.3 (2014): 499-520. Dolado, J. J., Ortigueira, S., & Stucchi, R. (2012). Does dual employment protection affect TFP? Evidence from Spanish manufacturing firms. Gash, V. (2008). Bridge or trap? Temporary workers’ transitions to unemployment and to the standard employment contract. European Sociological Review, 24(5), 651-668. Gerfin, M., Lechner, M., & Steiger, H. (2005). Does subsidised temporary employment get the unemployed back to work? Aneconometric analysis of two different schemes. Labour economics, 12(6), 807-835. Heckman, J. J., & Pages, C. (2003). Law and employment: Lessons from Latin America and the Caribbean. Holmes, T. J. (1998). The effect of state policies on the location of manufacturing: Evidence from state borders. Journal of political Economy, 106(4), 667-705. Hospido, L., & Moreno-Galbis, E. (2015). The Spanish productivity puzzle in the great recession. Hospido, L., & Moreno-Galbis, E. (2015). The Spanish productivity puzzle in the great recession. Kugler, A. D. (2004). The effect of job security regulations on labor market flexibility. Evidence from the Colombian Labor Market Reform. In Law and Employment: Lessons from Latin America and the caribbean (pp. 183-228). University of Chicago Press. Kugler, A., & Kugler, M. (2009). Labor market effects of payroll taxes in developing countries: Evidence from Colombia. Economic development and cultural change, 57(2), 335-358. Lazear, E. P. (1990). Job security provisions and employment. The Quarterly Journal of Economics, 105(3), 699-726. Lucidi, F. (2010, September). Labour market flexibility and productivity growth: new evidence from firm-level data. In XXIII National Conference of Labour Economics, Brescia (pp. 11-12). Micco, A., & Pagés, C. (2006, May). The economic effects of employment protection laws. In IZA/ The World Bank Conference on employment and development (pp. 25-27). OECD (2005) OECD economic surveys: Spain 2005. OECD, Paris Ortega, B., & Marchante, A. J. (2010). Temporary contracts and labour productivity in Spain: a sectoral analysis. Journal of Productivity analysis, 34(3), 199-212. Sanyal, P., & Menon, N. (2005). Labor disputes and the economics of firm geography: A study of domestic investment in India. Economic Development and Cultural Change, 53(4), 825-854. Taymaz, E. (2002, November). Are small firms really less productive? An analysis of productivity differentials and firm dynamics. In International Workshop on” The Post-entry Performance of Firms: Technology, Growth and Survival”, University of Bologna. Vidal, M., & Tigges, L. M. (2009). Temporary employment and strategic staffing in the manufacturing sector. Industrial Relations: A Journal of Economy and Society, 48(1), 55-72. Wang, R., & Weiss, A. (1998). Probation, layoffs, and wage-tenure profiles: A sorting explanation. Labour Economics, 5(3), 359-383. |